Fine-Tuning: The Ultimate Guide for Precision and Accuracy

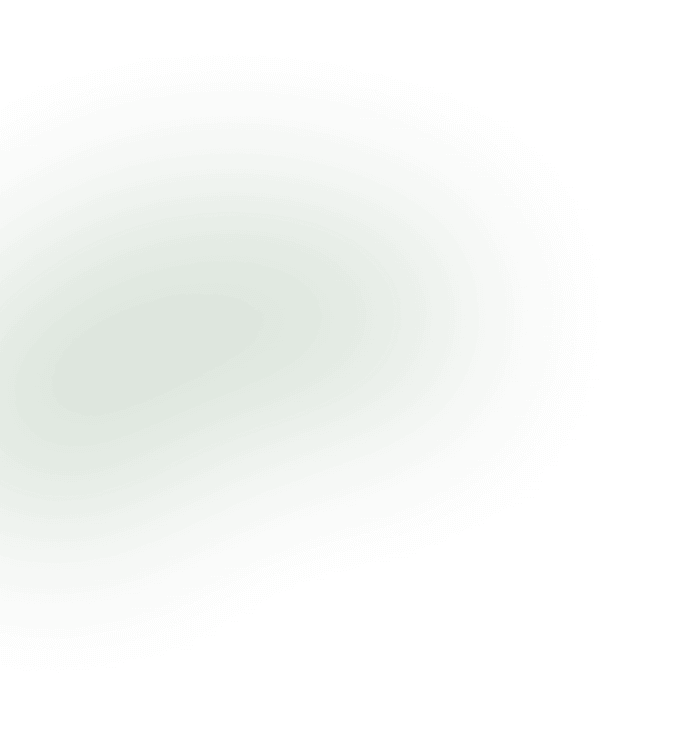
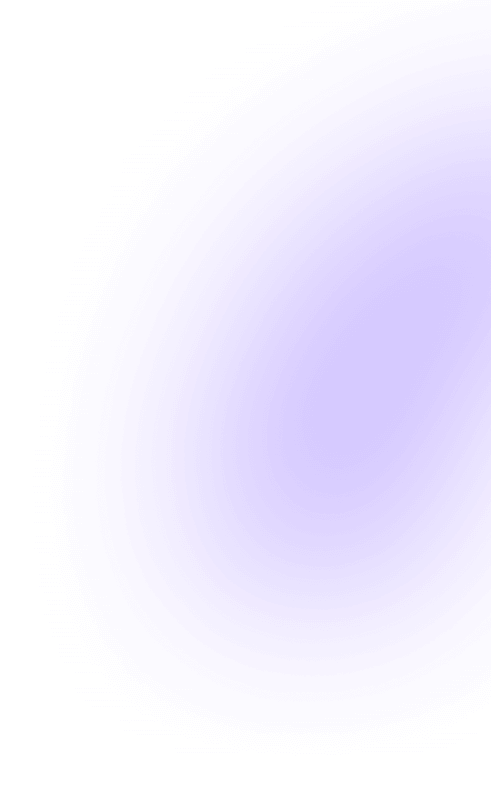
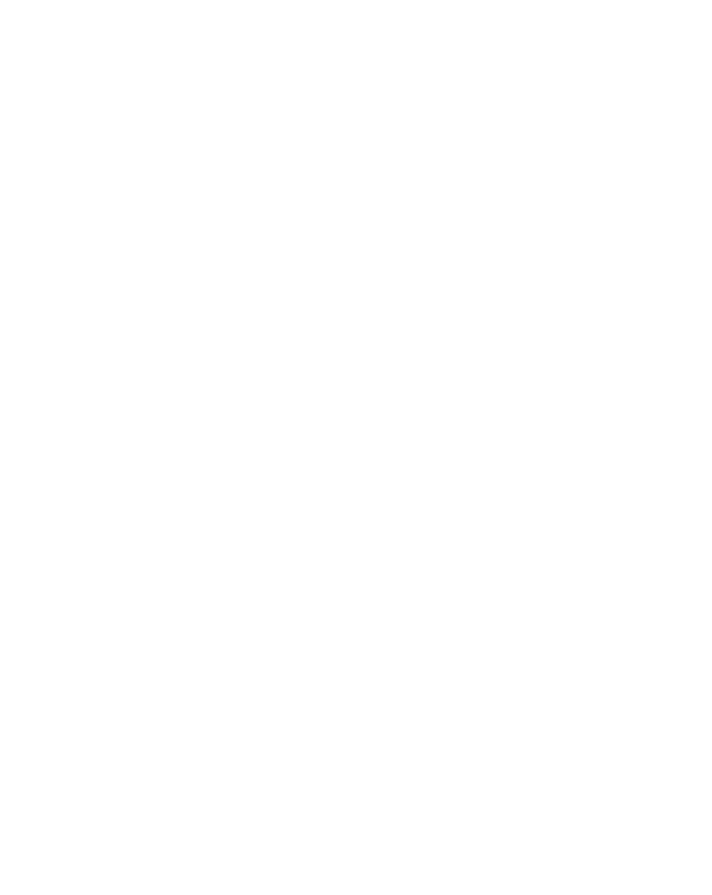
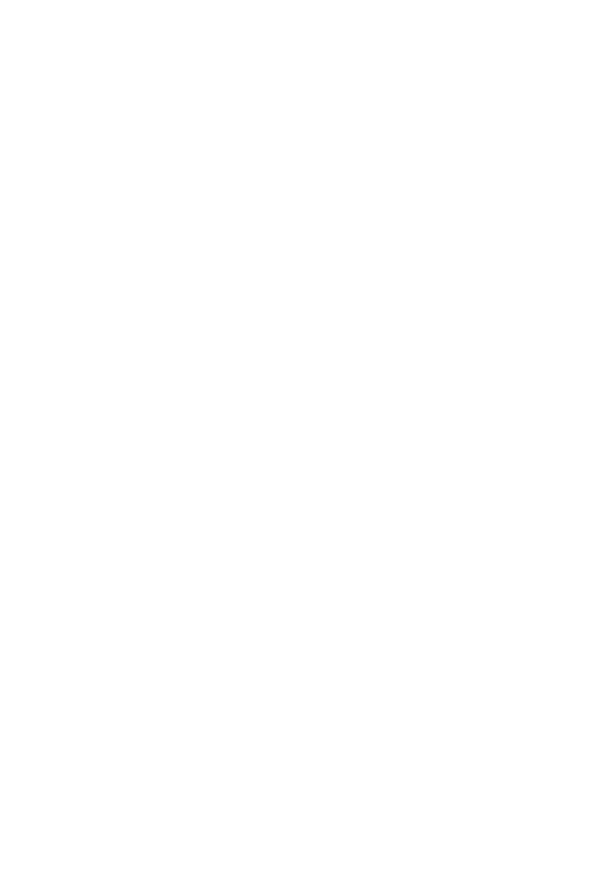
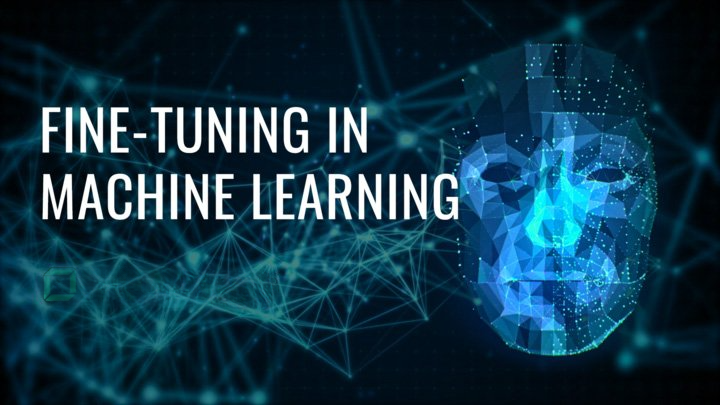
In the realm of technology and beyond, fine-tuning emerges as a pivotal process for enhancing performance, accuracy, and efficiency. This guide embarks on a journey to unravel the complexities and simplicities of fine-tuning, offering a comprehensive understanding of its essence and its paramount importance across various disciplines. From machine learning models to the precision in linguistic nuances, fine-tuning stands as the cornerstone of achieving unparalleled excellence.
The essence of fine-tuning lies in its ability to meticulously adjust and refine existing frameworks, models, or strategies to suit specific needs or to improve upon their original design. It is a deliberate and often intricate process that requires a deep understanding of the underlying system and the goals it seeks to achieve. In the context of machine learning, for example, fine-tuning involves the adjustment of parameters within a pre-trained model to enhance its performance on a new task.
Moreover, the concept of fine-tuning transcends the boundaries of technology, infiltrating daily tasks and personal development strategies. Whether it’s tweaking a recipe to perfection or adjusting a work routine for maximum productivity, the principles of fine-tuning apply universally, offering a path to optimization and excellence. The guide delves into these applications, showcasing the versatility and ubiquity of fine-tuning in improving outcomes.
Understanding the mechanism behind fine-tuning is crucial for harnessing its full potential. This involves not only recognizing the need for adjustments but also identifying the specific levers or parameters that can be modified to achieve the desired effect. In the technological sphere, this could mean altering the weights of a neural network, while in a personal context, it might involve modifying habits to foster growth and learning.
The comparative analysis between fine-tuning and transfer learning further illuminates the nuanced differences and complementary nature of these approaches. While both strategies aim to leverage existing knowledge or frameworks, fine-tuning focuses on refinement for specific tasks, whereas transfer learning emphasizes the application of learned knowledge to different but related tasks. This distinction is critical for selecting the appropriate method based on the objectives at hand.
Real-world applications and examples of fine-tuning abound, demonstrating its critical role in achieving precision and accuracy across fields. From the fine-tuning of algorithms that power recommendation systems to the subtle adjustments in communication strategies for effective leadership, the impact of fine-tuning is profound and far-reaching. This guide seeks to explore these examples, offering insights into how fine-tuning can be applied to solve complex problems and enhance outcomes in diverse scenarios.
The Essence of Fine-Tuning
At its core, fine-tuning represents a sophisticated process of making precise adjustments to a system, model, or methodology to achieve optimal performance or outcomes. It is an art and science that balances the intricate interplay between various elements within a system to enhance its functionality or efficiency. The essence of fine-tuning lies in its meticulous attention to detail and the pursuit of excellence through incremental improvements.
This process is not limited to any single discipline or field but is a universal principle that can be applied wherever there is a need for enhancement or customization. In the context of machine learning, it involves modifying an existing model to better perform a specific task. However, the principles of fine-tuning extend beyond technological applications, influencing areas such as personal development, business strategies, and everyday problem-solving. Through fine-tuning, individuals and organizations alike can achieve remarkable precision and accuracy, leading to superior results and advancements.
Understanding the Basics
Fine-tuning is grounded in the principle of starting with an existing foundation and making targeted adjustments to improve performance or adapt to new circumstances. This approach is particularly prevalent in fields where foundational models or frameworks can be applied across multiple contexts, with modifications enabling them to excel in specific tasks. The basic premise involves identifying the aspects of the system that can be optimized and implementing changes that refine its output or functionality.
At the heart of fine-tuning is the recognition that even well-designed systems can benefit from adjustments tailored to specific needs or goals. This perspective fosters a culture of continuous improvement and innovation, where the quest for excellence is ongoing. By focusing on the components that can be enhanced, fine-tuning enables significant gains in performance, often with relatively minor modifications.
The process begins with a thorough analysis of the existing system, including its strengths and limitations, followed by the identification of potential areas for improvement. From there, strategic adjustments are made, tested, and refined as necessary. This iterative process ensures that fine-tuning not only addresses immediate needs but also contributes to the long-term resilience and adaptability of the system.
The Mechanism Behind Fine-Tuning
The mechanism of fine-tuning involves a detailed and methodical approach to optimization. It starts with a comprehensive assessment of the existing system or model, identifying the specific components that require adjustments to meet new challenges or improve performance. This step is crucial for ensuring that the fine-tuning process is both effective and efficient, targeting areas that offer the greatest potential for enhancement.
In the context of machine learning, fine-tuning typically focuses on adjusting the parameters of a pre-trained model. These models, having already learned from vast amounts of data, possess a foundational understanding that can be refined for specific tasks. The fine-tuning process involves training the model on a new dataset, allowing it to adjust its weights and biases to better perform the task at hand. This method leverages existing knowledge, reducing the need for training from scratch and accelerating the path to high performance.
The success of fine-tuning hinges on a delicate balance between retaining the valuable insights gained from the original training and making sufficient adjustments to adapt to new data or objectives. This balance ensures that the model remains robust and versatile, capable of achieving high accuracy in a wide range of applications. Through careful analysis and strategic modifications, fine-tuning unlocks the full potential of existing models, enhancing their performance and extending their applicability.
Fine-Tuning vs. Transfer Learning: A Comparative Analysis
Fine-tuning and transfer learning are both pivotal in leveraging existing knowledge to address new problems, yet they differ in their approaches and objectives. Fine-tuning involves making subtle adjustments to a pretrained model to tailor it for a specific task. This process retains the structure and foundational knowledge of the model, focusing on optimizing it for enhanced performance on a given dataset. It is particularly effective in scenarios where the new task is closely related to the original training of the model.
Transfer learning, on the other hand, involves taking a model that has been trained on one task and repurposing it for a different but related task. This approach not only includes adjusting the model but often also entails modifying or expanding its architecture to accommodate the new task. Transfer learning is especially useful when the new task is significantly different from the original one, requiring the model to generalize its learned knowledge in novel ways. Both strategies harness the power of existing models to accelerate learning and improve outcomes, but they do so through distinct mechanisms tailored to the nature of the task at hand.
Real-World Applications and Examples
The application of fine-tuning spans a wide array of fields, demonstrating its versatility and effectiveness in enhancing performance and accuracy. In the realm of natural language processing (NLP), fine-tuned models have revolutionized language understanding, enabling systems to grasp nuances and context more adeptly than ever before. These models, refined through fine-tuning, offer improved responses and insights, significantly enhancing user experiences in applications such as virtual assistants and text analysis tools.
In computer vision, fine-tuning has led to breakthroughs in image recognition and classification. By adjusting pretrained models to focus on specific types of images or visual data, researchers and developers have achieved remarkable accuracy in tasks ranging from medical diagnosis to autonomous vehicle navigation. These fine-tuned models can identify patterns and details that are crucial for making accurate decisions based on visual inputs, showcasing the transformative power of fine-tuning in technology.
Moreover, fine-tuning has found applications beyond the technological sphere, influencing strategies in marketing, education, and personal development. By applying the principles of fine-tuning to these areas, individuals and organizations can optimize their approaches to better meet the needs and preferences of their audiences or achieve personal growth objectives. Whether it’s refining a marketing campaign or tailoring a learning plan, fine-tuning offers a path to enhanced outcomes through careful adjustment and optimization.
The Impact of Fine-Tuning
The impact of fine-tuning extends far beyond the immediate enhancements it brings to specific tasks or systems. By enabling more precise and accurate outcomes, fine-tuning contributes to the development of more efficient, effective, and user-friendly solutions across various domains. Its role in advancing technology and improving daily processes underscores the transformative potential of meticulous optimization.
Moreover, the practice of fine-tuning fosters a culture of continuous improvement and innovation. As individuals and organizations embrace the principles of fine-tuning, they are encouraged to constantly seek out opportunities for refinement and enhancement. This mindset not only leads to superior products and services but also promotes adaptability and resilience in the face of evolving challenges and opportunities.
Ultimately, the widespread adoption of fine-tuning has the potential to drive significant advancements in technology, business, and society. By prioritizing precision and accuracy, and by continually refining and adapting strategies and solutions, the impact of fine-tuning can be profound, paving the way for breakthroughs and improvements that enhance the quality of life and propel us toward a more efficient and effective future.
Advantages and Potential Risks
One of the major advantages of fine-tuning a model is the ability to leverage pre-existing machine learning capabilities. This approach allows for the utilization of a fine-tuned model that has been previously trained on vast amounts of data, making it more efficient and less resource-intensive than training a model from scratch. Consequently, this can lead to improved performance on specific tasks, as the model has already learned general features during its initial training phase.
However, despite these benefits, fine-tuning a model carries potential risks that must be considered. A significant risk is the possibility of overfitting, especially when the fine-tuning process is applied to a small dataset. Overfitting occurs when a model learns the training data too well, including its noise and outliers, which can negatively impact its performance on new, unseen data. Additionally, the quality of the training data plays a crucial role in the outcome of the fine-tuning process. If the training data contains biases, these can be inadvertently amplified by the model, leading to skewed results and decisions.
Another challenge lies in the balance between adapting the fine-tuned model to new tasks and retaining the knowledge it has previously acquired. This balance is crucial to avoid catastrophic forgetting, a scenario where the model forgets its initial training. Striking the right balance requires careful planning and testing to ensure that machine learning models remain generalizable and effective across various tasks.
Examples of Fine-Tune in Real Scenarios
One real-world application of fine-tuning can be observed in the realm of natural language processing (NLP), where machine learning models are fine-tuned to improve their understanding of context and nuances in human language. For instance, a model initially trained on a broad dataset of English language might be fine-tuned with specific legal or medical documents to adapt its capabilities for those sectors. This allows for more accurate predictions and analyses in fields where precision is paramount.
Another example is in image recognition tasks, where models pre-trained on generic image datasets are fine-tuned with specific datasets, such as x-rays or aerial photographs, to enhance their ability to identify relevant patterns and anomalies pertinent to particular disciplines. Such fine-tuning not only improves the model’s accuracy but also significantly reduces the time and resources required for training models from scratch for each unique application.
Solving Complex Problems with Precision
Fine-tuning a model allows for the tackling of complex problems with a high degree of precision by building upon pre-existing machine learning models. This method is particularly effective in domains where the nuances of the data play a critical role in the outcome, such as in predictive maintenance for industrial machinery. By fine-tuning models with specific operational data from the machinery, the models can predict failures more accurately, thereby preventing costly downtimes.
In the healthcare sector, fine-tuning is employed to enhance the diagnostic capabilities of machine learning models. Models pre-trained on general medical data can be fine-tuned with specific types of medical imagery, such as MRI scans, to detect anomalies with greater precision. This process significantly benefits patient care by enabling early detection of conditions that might not be evident to the human eye.
Moreover, fine-tuning facilitates the customization of machine learning solutions to meet the unique needs of different industries. By adjusting models to focus on specific characteristics of the data, organizations can solve complex problems that are tailored to their operational context. This adaptability is crucial for staying ahead in a rapidly evolving technological landscape, where the ability to quickly and accurately process and analyze data can provide a significant competitive advantage.
The Theory Behind the Practice
The theory underpinning fine-tuning in machine learning is grounded in the concept of transfer learning. This approach posits that knowledge gained while solving one problem can be applied to different but related problems. The essence of fine-tuning lies in adjusting the parameters of a pre-trained model so that it can learn from new data related to a specific task, thereby enhancing its performance on that task without having to learn from scratch.
Transfer learning, and by extension fine-tuning, challenges the traditional notion that machine learning models must be trained in isolation for each new task. Instead, it leverages the inherent patterns and features learned by models during their initial training on large datasets. This not only accelerates the training process for new tasks but also requires significantly less data, making it an efficient method for developing specialized applications.
The balance between retaining learned knowledge and adapting to new tasks is a fundamental aspect of fine-tuning theory. The process involves carefully adjusting the learning rate and deciding which layers of the model to freeze or train, based on the task at hand. This strategic manipulation of model parameters allows for the preservation of generalizable features while honing in on specifics of the new data, embodying the theory’s emphasis on efficiency and adaptability.
The Evolution and Word History of Fine-Tuning
The concept of fine-tuning has its roots in the early days of machine learning and has evolved significantly over the years. Initially, the term was used more broadly to describe the process of making small adjustments to improve the performance of a system. Over time, as machine learning capabilities advanced, fine-tuning came to specifically denote the practice of adjusting pre-trained models for enhanced performance on particular tasks.
The word history of “fine-tuning” reflects its broader applications beyond machine learning, including in physics, where it describes the precise adjustments needed to align with specific theoretical expectations. In the context of machine learning, fine-tuning encapsulates the nuanced process of adapting machine learning models to new, often more specialized, applications while building on the foundational knowledge these models have already acquired.
Cite This Entry: The Importance of Referencing
In the academic and professional world of machine learning, the act of referencing and citing sources is crucial for a multitude of reasons. Firstly, it lends credibility to the work, demonstrating that the conclusions drawn are backed by existing research and evidence. This is especially important in the field of machine learning, where the rapid pace of development means that new discoveries and methodologies are constantly emerging. Proper citation allows researchers and practitioners to trace the evolution of ideas and understand the context in which a fine-tuned model was developed.
Moreover, referencing facilitates the sharing of knowledge within the community, enabling others to build upon previous work. In the context of fine-tuning, citing the original models and datasets used as the foundation for further adjustments is essential for replicability and transparency. It allows others to understand the baseline from which improvements are made and to replicate the process for their purposes, thereby advancing the collective knowledge of the field.
Lastly, proper citation practices protect against plagiarism and intellectual property disputes. In machine learning, where proprietary algorithms and data can be invaluable, acknowledging the sources of pre-trained models and methodologies ensures respect for the original creators’ contributions. It fosters a culture of integrity and collaboration, which is vital for the continued growth and innovation in machine learning and its applications.
Enhancing Your Skills Through Fine-Tuning
Enhancing machine learning skills through fine-tuning involves a multifaceted approach that extends beyond the technical manipulation of algorithms. It requires a deep understanding of the data, the model’s architecture, and the specific task at hand. Practitioners must develop an intuition for how changes in the model’s parameters can affect its performance on different types of data. This includes recognizing when a model is overfitting and knowing how to adjust the training process to mitigate this issue.
Moreover, skill enhancement through fine-tuning also entails staying informed about the latest advancements in machine learning technologies and methodologies. Continuous learning and experimentation are key, as the field is rapidly evolving. Practitioners should engage with the wider machine learning community through forums, conferences, and collaborative projects to exchange knowledge and ideas.
Applying fine-tuning in practical projects is another effective way to enhance one’s skills. By working on diverse projects, practitioners can gain hands-on experience with various models, datasets, and challenges. This not only improves their technical abilities but also hones their problem-solving skills, making them more adept at applying machine learning models to real-world scenarios. Through this iterative process of learning, applying, and refining, practitioners can significantly advance their machine learning capabilities.
Practical Exercises to Master Fine-Tuning
One practical exercise to master fine-tuning involves working with a pre-trained model on a dataset different from the one it was initially trained on. For instance, starting with a model trained on general image recognition tasks, practitioners can fine-tune it on a specialized dataset, such as satellite images or medical scans. This exercise not only provides hands-on experience with fine-tuning techniques but also familiarizes practitioners with the intricacies of adapting models to new domains.
Another valuable exercise is participating in machine learning competitions, such as those hosted on platforms like Kaggle. These competitions often require participants to fine-tune pre-existing models to achieve the best performance on a given task. Competing offers a practical, goal-oriented way to apply fine-tuning techniques, encouraging practitioners to explore innovative approaches and learn from the strategies employed by their peers.
Can You Solve 4 Words Using Fine-Tuning Methods?
Applying fine-tuning methods to solve word puzzles presents an intriguing challenge that can sharpen one’s skills in pattern recognition and linguistic analysis. For instance, fine-tuning a model on a dataset comprising word puzzles and their solutions can enable it to learn the underlying patterns and strategies used to solve such puzzles. By iteratively training the model on increasing levels of difficulty, practitioners can explore how fine-tuning impacts the model’s ability to generalize and adapt to new puzzles.
This exercise can be extended by incorporating constraints, such as limiting the model to use only vowel-less words or to identify words based on scrambled letters. Such constraints force the model to focus on deeper linguistic structures and relationships, further enhancing its problem-solving capabilities. Practitioners can gain insights into the model’s learning process and identify areas for improvement, making the exercise both challenging and educational.
Finally, comparing the performance of fine-tuned models on different types of word puzzles can provide valuable lessons on the versatility and adaptability of machine learning models. Through these exercises, practitioners can not only improve their technical skills in fine-tuning but also develop a more nuanced understanding of the complexities involved in natural language processing and pattern recognition.
Fine-Tuning in Language and Communication
Language serves as the bedrock of human communication, evolving constantly to meet our ever-changing needs. Fine-tuning in language and communication, therefore, plays a crucial role in enhancing clarity, precision, and effectiveness. This process involves the meticulous adjustment of linguistic elements, from grammar and syntax to the nuanced use of vocabulary, ensuring messages are conveyed as intended. As language is a dynamic entity, adapting to new contexts and technologies, the importance of fine-tuning cannot be overstated. It bridges the gap between mere communication and impactful, meaningful interaction.
In the realm of digital communication, fine-tuning has gained new dimensions. The advent of generative AI and pretrained language models has revolutionized how we interact with text and speech online. These technologies rely on the fine-tuning of existing models, leveraging vast databases of language to understand and generate human-like responses. Such advancements underscore the significance of precision in language not just for humans but also for the machines that learn from our patterns of communication.
Moreover, the art of fine-tuning in language extends beyond the digital sphere into everyday life. Whether it’s drafting an email, engaging in conversation, or presenting an idea, the ability to adjust one’s language for clarity, tone, and context can dramatically affect the outcome. This aspect of language is not static but an ongoing process of learning and adaptation, highlighting the continuous nature of fine-tuning in both personal and professional growth.
Grammar & Usage: Sharpening Your Linguistic Tools
At the core of effective communication lies a solid grasp of grammar and usage. These foundational elements act as the tools through which ideas are sculpted and shared. Understanding the rules of grammar and their application not only prevents miscommunication but also enriches the language, allowing for greater expressiveness and precision. As such, fine-tuning one’s grasp of linguistic rules is akin to sharpening the very tools that construct meaning.
This process involves more than memorizing rules; it requires an active engagement with the language, identifying common pitfalls and learning how to avoid them. Through practice and exposure, individuals can refine their use of grammar, enhancing both their written and spoken communication. This level of linguistic fine-tuning not only bolsters one’s ability to convey complex ideas but also elevates the overall quality of interaction, making it more engaging and effective.
Your vs You’re: Avoiding Common Mistakes
Among the most common grammatical errors are the misuse of “your” and “you’re,” which, despite their similar pronunciation, serve distinct functions within the English language. “Your” denotes possession, indicating that something belongs to someone, whereas “you’re” is a contraction for “you are,” used to describe a state of being or action. Misuse of these terms can lead to confusion and detract from the clarity of communication.
To fine-tune one’s writing and avoid these errors, it is essential to understand the context in which each term should be used. This requires not only a grasp of grammar but also an attentiveness to the nuances of language. Practicing the correct usage of “your” and “you’re” in various sentences can help reinforce their distinctions, making it easier to avoid common mistakes.
Moreover, the misuse of “your” and “you’re” highlights the broader challenge of homophones in English, which requires a nuanced understanding beyond mere spelling. Fine-tuning one’s awareness of such grammatical nuances is a continuous process, essential for clear and effective communication. It reflects the ongoing nature of learning in language, where mastery is achieved through persistent effort and attention to detail.
Silent Letters and Absent Sounds: A Deep Dive
The English language is riddled with silent letters and absent sounds, a characteristic that often poses challenges for both native speakers and learners alike. These silent elements can alter the pronunciation of words without any indication from their spelling, making English particularly difficult to master. Understanding the role and patterns of silent letters is crucial for fine-tuning pronunciation and enhancing linguistic accuracy.
For instance, the silent “k” in “knife” or the absent sound in the middle of “Wednesday” require a deeper understanding of English phonetics and etymology. This knowledge not only aids in pronunciation but also enriches one’s comprehension of the language’s history and evolution. Fine-tuning one’s awareness of these silent elements involves a blend of memorization, practice, and exposure to a wide range of texts and spoken language.
Ultimately, mastering the intricacies of silent letters and absent sounds exemplifies the fine-tuning process in language learning. It demands patience, attention to detail, and an appreciation for the complexity of linguistic structures. By embracing these challenges, individuals can achieve greater precision in their communication, reflecting the nuanced and dynamic nature of language itself.
The Art of Wordplay and Fine-Tuning
Wordplay represents a sophisticated level of linguistic finesse, showcasing the playful yet profound capability of language to entertain and engage. The art of wordplay, from puns and anagrams to complex literary devices, illustrates the power of fine-tuning in crafting messages that are not only clear but also creatively stimulating. This linguistic creativity demands a deep understanding of language’s rules and the flexibility to bend them in clever, unexpected ways.
The effectiveness of wordplay lies in its ability to alter perspectives, challenge expectations, and evoke a wide range of emotions. Whether in literature, advertising, or casual conversation, fine-tuning one’s use of wordplay can significantly enhance the impact of communication. It requires a balance between precision and inventiveness, demonstrating how strategic language use can transform ordinary messages into memorable experiences.
Moreover, the practice of wordplay encourages a playful exploration of language, inviting individuals to experiment with words and meanings. This aspect of fine-tuning not only enriches personal expression but also fosters a deeper connection with the audience. By engaging in the art of wordplay, communicators can unlock new dimensions of language, revealing its capacity to delight, persuade, and inspire.
From Scrabble to Insults: The Role of Fine-Tuning in Word Games
The realm of word games, from Scrabble to witty exchanges of insults, serves as a testament to the importance of fine-tuning in language. These games challenge participants to meticulously choose their words, balancing the need for precision with the desire for creativity. In Scrabble, for example, the ability to spot potential word combinations within a limited set of letters requires not only a vast vocabulary but also the strategic fine-tuning of linguistic possibilities.
Similarly, the artful crafting of insults, especially in a playful context, demands a keen understanding of language’s power and subtlety. It involves fine-tuning one’s choice of words to achieve the desired effect, whether to amuse, disarm, or demonstrate wit. This aspect of wordplay highlights the dynamic interplay between meaning and expression, where the precise calibration of language leads to engaging and memorable encounters.
10 Vowel-less Scrabble Words: A Challenge
Scrabble enthusiasts often relish the challenge of crafting words under constraints, and one of the most intriguing puzzles involves creating words without vowels. These vowel-less words not only test players’ vocabulary but also their ability to fine-tune their strategy within the game’s rules. Examples of such words include “rhythms,” “brrr,” “tsktsks,” “nymph,” “gypsy,” “pyx,” “pfft,” “sh,” “byrls,” and “crypt.” Each word showcases the flexibility and depth of English, pushing players to explore beyond conventional word structures.
The ability to recognize and utilize vowel-less words in Scrabble demonstrates the critical role of fine-tuning in language mastery. It requires a deep dive into the less trodden paths of vocabulary, uncovering words that, despite their rarity or complexity, are legitimate and valuable in play. This skill not only enhances one’s game strategy but also enriches linguistic knowledge, revealing the vast potential of language when constraints force creativity.
Moreover, engaging with vowel-less words in Scrabble encourages players to fine-tune their understanding of language rules and exceptions. It exemplifies how language, even within the framework of a game, is a dynamic system of communication, full of surprises and learning opportunities. Such challenges underscore the joy and complexity of language, inviting players to continuously explore and refine their linguistic skills.
Bird Names and Superb Owls: Exploring Linguistic Creativity
The exploration of bird names offers a fascinating opportunity to delve into linguistic creativity and the art of fine-tuning. Bird names, with their often whimsical and evocative qualities, serve as a rich source of inspiration for wordplay, including puns like “Superb Owl” for “Super Bowl.” Such playful manipulation of language not only entertains but also highlights the nuanced ways in which words can be reimagined and repurposed.
Engaging with bird names through the lens of wordplay encourages a deeper appreciation for the diversity and beauty of language. It invites individuals to fine-tune their perception of words, recognizing the potential for creativity within everyday vocabulary. This practice not only enhances one’s linguistic agility but also fosters a connection with the natural world, as the names of birds often reflect their characteristics or the environments they inhabit.
Ultimately, the exploration of bird names and the creation of wordplay like “Superb Owls” exemplify the endless possibilities within language. It demonstrates how fine-tuning one’s approach to words can reveal layers of meaning and humor, enriching both personal expression and collective understanding. By embracing the inherent creativity of language, individuals can discover new ways to engage with and appreciate the world around them.
Leveraging Fine-Tuning for Better Outcomes
Fine-tuning, in the context of machine learning and artificial intelligence, has emerged as a pivotal strategy for enhancing the performance of pre-trained models. By adjusting a model’s parameters slightly, developers can adapt it to fulfill specific tasks, leading to better outcomes than would be possible by training a model from scratch. This process not only saves significant resources but also leverages existing knowledge embedded within the model, making it a preferred choice for many applications.
Real-world examples abound where fine-tuning has led to groundbreaking advancements. In industries ranging from healthcare to finance, the ability to customize machine learning models to address unique challenges has led to more accurate predictions, personalized customer experiences, and efficient data processing. This adaptability underscores the practical value of fine-tuning, as it enables organizations to harness the power of AI more effectively.
The technology sector, in particular, has benefitted from the application of fine-tuning. By refining pre-trained models, companies have been able to develop innovative solutions that push the boundaries of what machine learning can achieve. These advancements not only highlight the technical capabilities of fine-tuned models but also illustrate the potential for these models to drive progress across various fields.
Real-life Examples Where Fine-Tuning Made a Difference
In healthcare, fine-tuning has revolutionized the way medical images are interpreted. By adjusting pre-trained models to better recognize specific patterns, doctors can now diagnose conditions more accurately and swiftly. Similarly, in the realm of natural language processing (NLP), fine-tuned models have significantly improved the quality of machine translation and sentiment analysis, making global communication more seamless and accurate.
The finance sector has seen similar benefits from fine-tuning. Customized models have enhanced fraud detection systems, enabling banks to identify suspicious activities with greater precision. This not only protects consumers but also reduces financial losses. In customer service, fine-tuned chatbots provide personalized assistance, improving customer satisfaction and operational efficiency.
Environmental science has also leveraged fine-tuning for better outcomes. By fine-tuning models to predict climate patterns, researchers can now provide more accurate forecasts, aiding in disaster preparedness and resource management. These examples underscore the transformative impact of fine-tuning across various domains, highlighting its role in solving complex problems with precision.
Fine-Tuning in Technology: Beyond the Basics
Fine-tuning extends beyond mere parameter adjustment; it represents a sophisticated approach to enhancing machine learning capabilities. In technology, this process involves training a pre-existing model on a new, typically smaller, dataset. This method not only refines the model’s understanding of specific tasks but also incorporates knowledge from diverse domains, enriching its learning capacity.
The evolution of fine-tuning techniques has enabled more nuanced modifications to models, allowing for the customization of layers and learning rates. This granularity ensures that the fine-tuning process can be tailored to the unique requirements of each task, further optimizing performance. As a result, technology companies can deploy highly specialized machine learning models, capable of tackling intricate challenges with unprecedented accuracy.
Moreover, the application of fine-tuning in technology has facilitated the development of innovative products and services. From personalized recommendation systems to sophisticated speech recognition software, fine-tuning has been instrumental in refining the capabilities of machine learning models. This has not only accelerated the pace of technological advancement but also opened up new avenues for applying AI in real-world scenarios.
Navigating the Risks and Rewards
The process of fine-tuning machine learning models presents a balance of risks and rewards. On one hand, the ability to customize and improve pre-trained models offers significant advantages in terms of cost, efficiency, and performance. Organizations can leverage existing models to achieve better results with less data and computational resources. On the other hand, fine-tuning requires careful management to avoid overfitting, where a model performs well on training data but poorly on new, unseen data.
Moreover, the reliance on pre-trained models can also introduce biases present in the original training data. If not addressed, these biases can perpetuate and even amplify undesirable outcomes. Therefore, while fine-tuning opens up new possibilities for enhancing machine learning models, it necessitates a critical approach to ensure that the models remain fair, accurate, and generalizable to new tasks and datasets.
Ultimately, the successful application of fine-tuning depends on a strategic balance. By recognizing the potential pitfalls and implementing measures to mitigate them, organizations can harness the benefits of fine-tuning while minimizing its risks. This strategic approach enables the development of machine learning solutions that are not only powerful and efficient but also responsible and equitable.
Analyzing the Benefits with a Critical Eye
The benefits of deploying a fine-tuned model are manifold, particularly when compared to developing a model from scratch. Fine-tuning allows for leveraging the vast knowledge already captured within pre-existing models, significantly reducing the time and resources required for training. This efficiency is especially valuable in scenarios where data is scarce or expensive to obtain. By utilizing a smaller set of training data, fine-tuned models can achieve high levels of accuracy and performance, tailored to specific tasks.
However, the advantages of fine-tuning extend beyond mere efficiency. Fine-tuned models often exhibit superior performance in specialized applications, as they can be meticulously adjusted to the nuances of the task at hand. This precision enables more effective solutions to complex problems, enhancing the potential for innovation and progress within various fields.
Despite these benefits, it’s crucial to approach fine-tuning with a critical eye. The process depends heavily on the quality of the underlying model and the relevance of the original training data to the new task. Misalignment between the pre-trained model’s knowledge and the target application can lead to suboptimal outcomes, underscoring the importance of careful model selection and adjustment.
Mitigating the Risks Through Strategic Approaches
To mitigate the risks associated with fine-tuning, a strategic approach is essential. One key strategy is the careful selection of training data. Ensuring that the data is representative of the new task can prevent overfitting and improve the generalizability of the fine-tuned model. Additionally, continuous monitoring and validation against unseen data sets are crucial for maintaining model accuracy over time.
Another important consideration is addressing biases within the pre-trained model. By identifying and correcting biases in the training data, developers can reduce the risk of perpetuating or amplifying these biases through the fine-tuned model. This not only improves the fairness and reliability of the model but also enhances its applicability to a wider range of scenarios.
Finally, a balanced approach to model complexity and customization can help optimize the fine-tuning process. While it’s tempting to extensively modify a pre-trained model, overly complex adjustments can lead to overfitting and reduced model interpretability. By focusing on targeted, meaningful adjustments, developers can achieve the benefits of fine-tuning while minimizing its risks, leading to more robust and effective machine learning solutions.
Fine-Tuning in Everyday Life
Fine-tuning principles extend beyond the realms of technology and science, finding applications in everyday life. The concept of making small, precise adjustments to improve performance or efficiency can be applied to various tasks, from personal time management to learning new skills. By adopting a mindset of continuous improvement, individuals can enhance their productivity and effectiveness in both personal and professional contexts.
In personal development, for example, fine-tuning might involve adjusting habits or routines to better align with one’s goals and values. This could mean refining a workout regimen for optimal health benefits or tweaking a study schedule to improve learning outcomes. The key is in the incremental adjustments that, over time, lead to significant improvements.
Similarly, in the professional sphere, businesses can apply fine-tuning strategies to optimize processes, improve customer experiences, and innovate products and services. Whether it’s refining a marketing strategy based on customer feedback or adjusting operational procedures for efficiency, the principles of fine-tuning can help organizations stay competitive and responsive to changing market demands. Through continuous evaluation and adjustment, fine-tuning enables both individuals and organizations to achieve better outcomes and foster growth.
Applying Fine-Tuning Principles to Daily Tasks
Incorporating fine-tuning principles into daily tasks starts with the recognition of patterns and the identification of areas for improvement. Whether it’s optimizing your morning routine to save time or adjusting your communication style for better clarity, fine-tuning encourages a mindset of ongoing refinement. It involves critically assessing each component of a task, no matter how mundane, and asking how it can be performed more efficiently or effectively. This iterative process of evaluation and adjustment can lead to significant enhancements in both productivity and personal satisfaction.
At the heart of applying fine-tuning to daily activities is the concept of marginal gains, the idea that small, incremental improvements cumulate into substantial progress over time. By focusing on making slight adjustments to habits and routines, individuals can slowly but surely transform their day-to-day experiences. This might mean reorganizing your workspace for better ergonomics or fine-tuning your budgeting techniques to better manage finances, each small adjustment building towards a more optimized lifestyle.
Moreover, the discipline learned through fine-tuning daily tasks transcends into other areas of life, fostering a culture of continuous improvement. As one becomes more adept at identifying inefficiencies and implementing solutions, this skill set can be applied to more complex challenges, both personal and professional. Consequently, the practice of fine-tuning can evolve from simple task management to a comprehensive strategy for life optimization.
The Impact on Personal and Professional Growth
The practice of fine-tuning can have profound implications for personal and professional growth. By continuously seeking ways to improve processes and outcomes, individuals cultivate a growth mindset that is vital for long-term success. This approach not only enhances one’s ability to adapt to change but also fosters a proactive stance towards learning and development. In the professional realm, for instance, fine-tuning skills such as time management and project planning can lead to more efficient work flows and higher quality outcomes, thereby accelerating career advancement.
On a personal level, the benefits of fine-tuning extend to greater self-awareness and fulfillment. By systematically addressing areas for improvement, individuals can achieve a better understanding of their strengths and weaknesses. This introspective process can lead to more informed decisions about career paths, hobbies, and relationships, enriching one’s life experience. Moreover, the habit of fine-tuning encourages resilience, as individuals learn to view setbacks not as failures but as opportunities for further refinement.
Furthermore, the practice of fine-tuning aligns closely with the principles of lifelong learning. As individuals strive for continual improvement, they naturally seek out new knowledge and experiences, enhancing their competencies and adaptability. This insatiable curiosity not only enriches their personal lives but also makes them invaluable assets in any professional setting, where innovation and flexibility are increasingly prized.
The Future of Fine-Tuning
The future of fine-tuning is poised at the intersection of technology and human ingenuity, where advancements in artificial intelligence (AI) and machine learning (ML) are expanding the boundaries of what can be optimized. As algorithms become more sophisticated, the capacity for fine-tuning complex systems and processes is significantly enhanced. This suggests a future where personalized learning paths, tailored healthcare treatments, and optimized business strategies become the norm, all powered by fine-tuning algorithms that learn and adapt over time.
Moreover, the role of fine-tuning in fostering sustainability and efficiency across industries cannot be overstated. As global challenges such as climate change and resource scarcity intensify, the need for finely-tuned solutions that maximize efficiency while minimizing waste becomes critical. This could manifest in smarter urban planning, more efficient food systems, and cleaner energy production, all benefiting from the principles of fine-tuning to create sustainable outcomes.
Additionally, the democratization of fine-tuning tools and techniques promises to empower individuals and organizations alike. With access to powerful analytics and AI-driven insights, small businesses, educators, and even individuals can apply fine-tuning principles to achieve remarkable improvements. This trend towards widespread accessibility suggests a future where the benefits of fine-tuning are not confined to elite institutions or corporations but are shared broadly, contributing to a more equitable and efficient world.
Emerging Trends and Predictions
Emerging trends in fine-tuning point towards an increasingly personalized and predictive approach across various domains. In education, for example, fine-tuning algorithms could enable highly customized learning experiences that adapt to each student’s unique needs and pace, a concept supported by initial training models in academic research. Similarly, in healthcare, predictive analytics, informed by fine-tuning, could revolutionize patient care by anticipating health issues before they become critical, thus enabling preventative interventions.
In the realm of technology, prompt engineering emerges as a critical component of fine-tuning natural language processing (NLP) systems. This practice involves crafting inputs that guide AI models to generate more accurate, relevant, and contextually appropriate outputs. As AI becomes more integrated into daily life, the importance of fine-tuning through prompt engineering to ensure these technologies are beneficial, ethical, and user-friendly cannot be overstated.
Further, the collaboration between humans and AI in the fine-tuning process is expected to deepen. This symbiosis will likely lead to the development of more intuitive interfaces and feedback mechanisms, where AI systems learn directly from human interaction, leading to more effective and personalized outcomes. Such advancements highlight the potential of fine-tuning not just as a technical tool but as a bridge between human creativity and computational power, unlocking new possibilities for innovation and problem-solving.
The Continued Relevance in a Changing World
In a rapidly evolving global landscape, the principles of fine-tuning remain more relevant than ever. As societal, technological, and environmental challenges become more complex, the ability to adapt and optimize solutions becomes crucial. Fine-tuning offers a framework for continuous improvement, essential for navigating the uncertainties of the 21st century. Whether it’s adjusting business strategies to meet changing market demands or refining personal habits for better health and well-being, fine-tuning provides the agility needed to thrive in a changing world.
The enduring relevance of fine-tuning is also evident in its application to sustainability efforts. As the world grapples with the effects of climate change, fine-tuning approaches to energy consumption, waste management, and resource allocation become vital. By applying fine-tuning principles, societies can develop more sustainable practices that not only address immediate needs but also safeguard the environment for future generations.
Moreover, the increasing complexity of technology and data underscores the importance of fine-tuning in ensuring ethical and effective use. As AI systems and big data analytics become entrenched in every aspect of life, from governance to personal finance, the need for fine-tuning to ensure these technologies serve the public good becomes paramount. Thus, fine-tuning stands as a critical practice in crafting solutions that are not only efficient and innovative but also equitable and sustainable.
Sharpening the Edge: Conclusion on Fine-Tuning
As we look to the future, the importance of fine-tuning in achieving precision, efficiency, and adaptability cannot be underestimated. It represents not just a technique for optimization, but a mindset of continuous improvement and innovation. In a world characterized by rapid change and complex challenges, the ability to fine-tune processes, technologies, and even personal habits is an invaluable asset. It enables individuals and organizations to remain competitive, resilient, and responsive to the evolving demands of the global landscape.
The journey of fine-tuning is one of exploration and refinement, where each iteration brings us closer to our goals. Whether in the pursuit of professional excellence, personal growth, or societal advancement, fine-tuning offers a path forward. It encourages us to question, to experiment, and to learn from each adjustment, fostering a culture of curiosity and resilience that is essential for the 21st century.
In conclusion, embracing fine-tuning is about more than seeking efficiency; it’s about cultivating a proactive approach to life and work. By continuously seeking opportunities for improvement, we not only enhance our immediate environment but also contribute to a broader culture of innovation and excellence. As we move forward, let us carry the principles of fine-tuning with us, applying them not only to the tasks at hand but to the broader challenges that lie ahead.
The Path Forward with Fine-Tuning
The future beckons with opportunities for those ready to embrace the principles of fine-tuning. As we navigate the complexities of modern life, the practice of continuously refining our approaches offers a roadmap to success. It’s about leveraging every tool, insight, and experience at our disposal to craft more effective, efficient, and adaptable strategies. This commitment to improvement will be key in addressing the multifaceted challenges of our time, from technological advancements to societal shifts.
Moreover, as the landscape of our world continues to evolve, the need for fine-tuning becomes ever more critical. By adopting a mindset that views change not as a hurdle but as an opportunity for refinement, we position ourselves to not only survive but thrive. The path forward with fine-tuning is one of endless potential, where each small adjustment can lead to significant breakthroughs. It’s a journey of continuous discovery, learning, and growth, driven by the unwavering pursuit of excellence.
Embracing Continuous Improvement and Precision
Embracing continuous improvement and precision through fine-tuning is a powerful strategy for personal and professional development. This approach fosters a culture of excellence, where the quest for betterment is ongoing. By applying fine-tuning principles, individuals and organizations can achieve remarkable levels of precision, enhancing performance and outcomes. This process of continual refinement is crucial in a world where standards and expectations are ever-rising, ensuring that we remain at the forefront of innovation and efficiency.
The practice of continuous improvement is not without its challenges, yet it is precisely these challenges that drive innovation and progress. Through the meticulous process of fine-tuning, we learn to identify and address the subtle nuances that can make a significant difference in the quality of our work and lives. This dedication to precision not only leads to superior results but also cultivates a mindset that values detail, accuracy, and excellence.
Ultimately, the journey towards embracing continuous improvement and precision is one of empowerment. It equips us with the skills and mindset necessary to navigate the complexities of the modern world, turning obstacles into opportunities for growth. As we continue to fine-tune our approaches, we not only enhance our own capabilities but also contribute to a broader culture of excellence and innovation. This commitment to refinement and precision is what will propel us forward, ensuring our relevance and success in an ever-changing landscape.