Exploring the Intricacies of Retrieval-Augmented Generation (RAG) AI

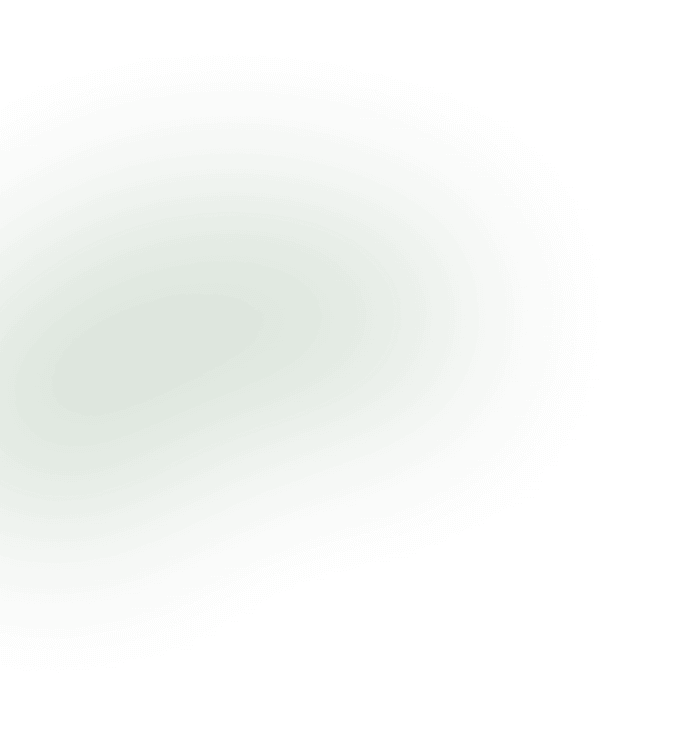
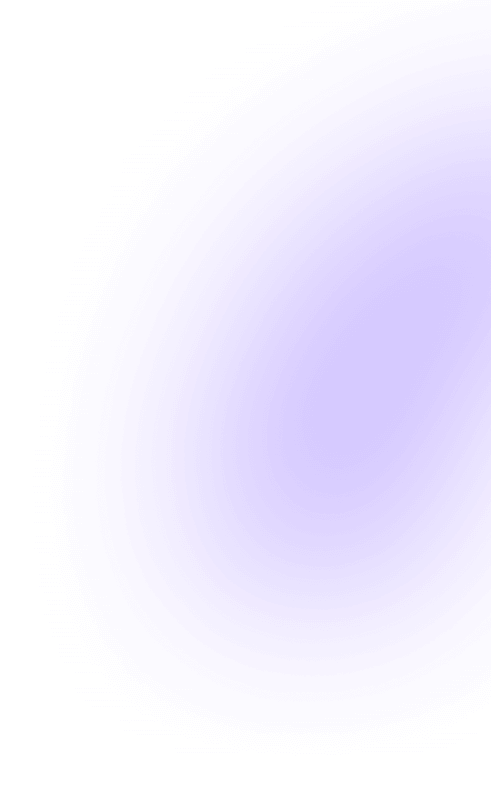
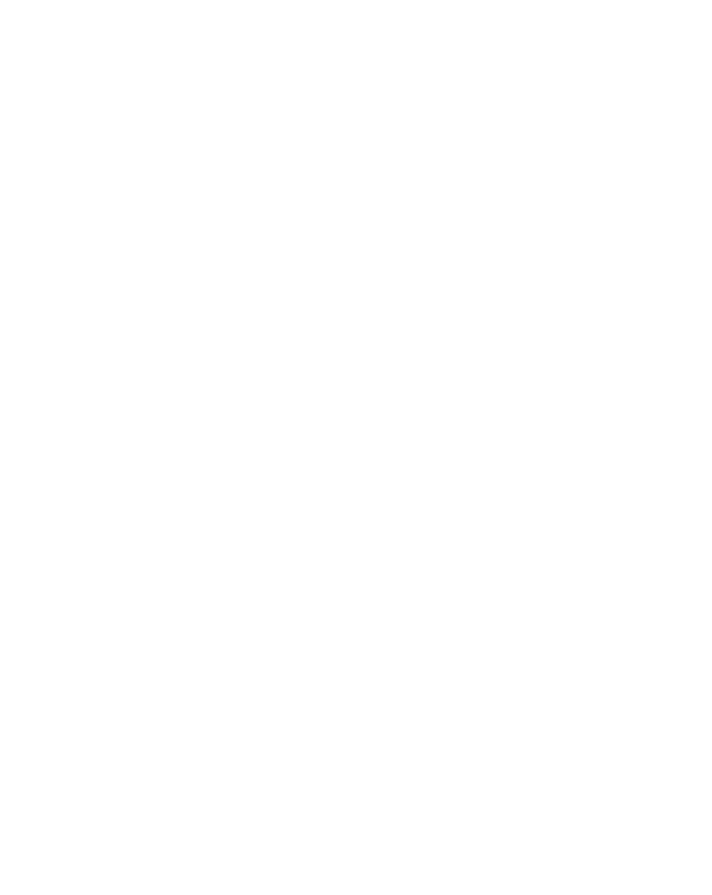
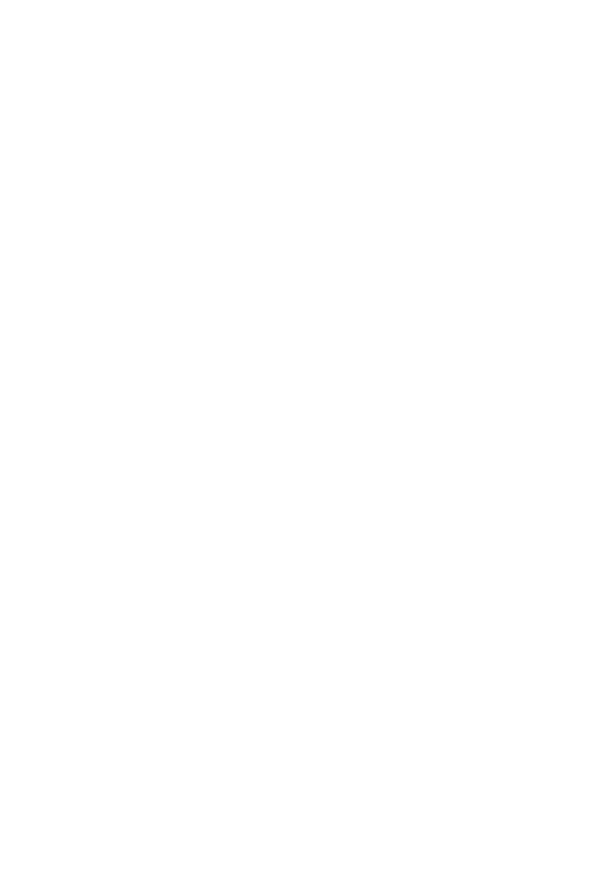
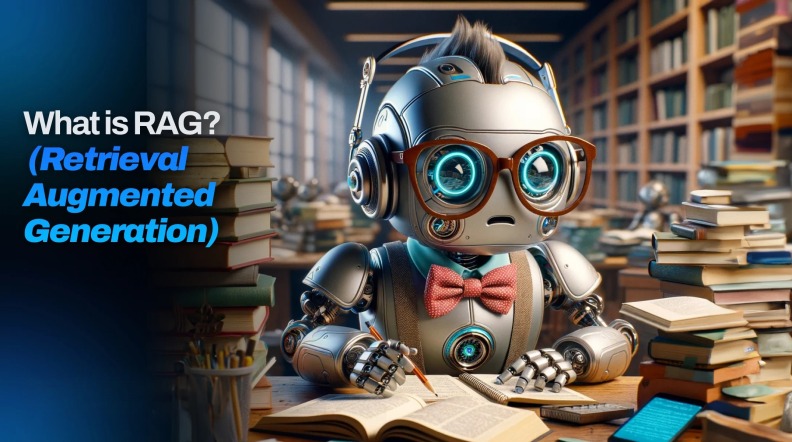
Retrieval-Augmented Generation (RAG) AI represents a significant evolution in the field of artificial intelligence, particularly within natural language processing (NLP). By enhancing the accuracy of responses through a symbiotic relationship between retrieval phases and generative capabilities, RAG AI introduces a dynamic approach to information processing. This methodology allows AI to pull facts from an external database, enriching the internal representation of knowledge before generating responses.
Central to RAG AI’s innovation is its ability to harness vast stores of raw data, transforming them into factually accurate and contextually relevant outputs. The 2020 paper that introduced RAG AI to a broader audience underscored its potential in tackling knowledge-intensive tasks, which demand not just accurate results but a deep understanding of context and content. This capability marks a departure from previous models that could generate plausible but often inaccurately inferred information.
RAG allows LLMs (Large Language Models) to exceed their inherent limitations by integrating external databases into the generative process. This integration enables the AI to consult up-to-date information, ensuring the relevance and accuracy of its outputs. AI researchers are particularly excited about RAG models for their ability to seamlessly merge the breadth of generative models with the depth of retrieval-based systems, presenting a holistic approach to AI-driven query resolution.
Unraveling the Definition and Importance of RAG AI
Retrieval-Augmented Generation (RAG) AI is a hybrid model that combines the depth of retrieval-based information gathering with the creative flexibility of generative AI systems. This amalgamation not only enhances the accuracy of generated content but also significantly expands the scope of AI’s applicability across various domains. The importance of RAG AI lies in its ability to produce more informed, relevant, and contextually nuanced responses than ever before.
The Essence of Retrieval-Augmented Generation
At the core of Retrieval-Augmented Generation is the principle of enhancing the accuracy of AI-generated content by integrating external data during the generation process. This approach ensures that the outputs are not only coherent and contextually appropriate but also enriched with the most current and relevant information available. The essence of RAG AI, therefore, lies in its capacity to bridge the gap between raw data and meaningful, actionable information.
Bridging the Gap Between Generative and Retrieval-Based Models
The advent of RAG AI has been pivotal in merging the capabilities of generative and retrieval-based models, creating a synergistic effect that leverages the strengths of both approaches. By accessing external knowledge sources, RAG AI enriches the generative process, ensuring that the information it produces is both accurate and comprehensive. This integration addresses the limitations of purely generative models, which can often generate plausible but uninformed content.
Furthermore, the inclusion of external knowledge sources allows for a dynamic updating mechanism within the generative process, providing AI systems with the ability to adapt to new information rapidly. This capability is critical for applications requiring up-to-the-minute accuracy, from financial analysis to healthcare diagnostics, highlighting the transformative potential of RAG AI in bridging the knowledge gap.
Why RAG AI Marks a Revolution in Technology
Retrieval-Augmented Generation (RAG) AI signifies a technological revolution by fundamentally altering how machines understand and interact with human language. It transcends traditional barriers between data retrieval and generation, offering a novel paradigm where AI can access, interpret, and incorporate external data in real-time. This advancement heralds a new era of AI capabilities, promising unprecedented levels of interaction and understanding.
The Need for Combining External Data with AI Capabilities
The integration of external data with AI capabilities, as exemplified in RAG AI, addresses a critical need in the field of artificial intelligence: the requirement for systems to not only generate content but to do so with a level of understanding and relevance that mimics human cognitive processes. Leveraging external data allows for general-purpose fine-tuning of AI models, enabling them to perform a wider array of tasks with greater accuracy and nuance.
Meta AI’s role in this integration process is pivotal, as it provides the frameworks and tools necessary for AI to access and utilize external data effectively. This capability ensures that AI systems are not limited by their pre-existing knowledge bases but can instead continuously expand their understanding through real-time data retrieval. Such advancements have profound implications for the development of AI, setting the stage for more adaptive, intelligent, and context-aware systems.
A Deep Dive into How RAG AI Functions
Understanding the functionality of Retrieval-Augmented Generation (RAG) AI involves a deep dive into its unique process, which seamlessly blends data retrieval with generative capabilities. This dual-phase operation allows AI systems to pull in relevant external data as a foundational step before embarking on the task of generating responses, thereby ensuring that the outputs are both informed and pertinent.
The Mechanics Behind RAG AI
The mechanics of RAG AI revolve around a two-step process: the retrieval phase and the generation phase. Initially, the system searches a vast repository of external data to find information relevant to the query at hand. This retrieval phase is critical for gathering the necessary context and details that will inform the subsequent generation of responses, setting RAG AI apart from traditional generative models.
Incorporating External Knowledge Sources for Enhanced Responses
The incorporation of external knowledge sources is a defining characteristic of RAG AI, enabling the system to produce responses that are not only contextually appropriate but also enriched with the latest information. This process involves querying databases, webpages, and other digital repositories during the retrieval phase, ensuring that the AI has access to a broad spectrum of data.
Once the relevant data is retrieved, RAG AI synthesizes this information to generate responses that are both accurate and relevant. This blending of retrieved data with the AI’s internal processing capabilities exemplifies the power of external knowledge sources in enhancing AI-generated content, making RAG AI a cornerstone technology for future applications across numerous fields.
The Role of Large Language Models in RAG AI
Large Language Models (LLMs) play a crucial role in RAG AI, particularly in the realm of NLP applications. These models, trained on vast datasets, provide the foundation upon which RAG AI builds, leveraging their generative capabilities to produce nuanced and contextually rich responses. The integration of LLMs in RAG AI systems is essential for understanding and processing human language at a level that approaches natural conversation.
From User Input to Contextualized Output: The Path of Data in RAG AI
The journey from user input to contextualized output in RAG AI involves a sophisticated interplay between retrieval systems and vector databases. Initially, the system employs a retrieval system to parse the user’s query and identify the key elements that will guide the search for relevant information. This involves scanning vector databases to retrieve relevant documents that match the query’s intent and context.
Following the retrieval of pertinent data, RAG AI integrates this information with its internal generative processes to construct responses that are tailored to the user’s original query. This pathway from raw user input to refined, contextualized output showcases the intricate mechanics of RAG AI, highlighting its ability to transform abstract queries into concrete, informative answers.
The Unseen Power of External Data in RAG AI
The integration of external data sources into RAG AI systems unveils a dimension of AI potential that was previously untapped. By accessing real-time information from diverse databases and digital repositories, RAG AI enriches its knowledge base, enabling more accurate and relevant responses. This unseen power of external data is the cornerstone of RAG AI’s ability to stay current and provide outputs that reflect the latest developments and insights.
Moreover, the use of external data sources facilitates a level of learning and adaptability that is unparalleled in traditional AI models. As RAG AI systems continuously access and integrate new information, they evolve, becoming more sophisticated and capable over time. This dynamic approach to knowledge acquisition and application positions RAG AI as a transformative force in artificial intelligence, poised to redefine the landscape of machine learning and NLP.
Harnessing APIs, Webpages, and Real-Time Databases
The ability of RAG AI to harness APIs, webpages, and real-time databases for data retrieval is a critical aspect of its operation. This access allows RAG AI to pull in a wide range of information, from the latest news articles to scientific research findings, ensuring that the generated responses are both current and comprehensive. The strategic use of these diverse data sources is what enables RAG AI to maintain its edge in generating informed and accurate content.
The Journey from Data Retrieval to Information Integration
The journey from data retrieval to information integration in RAG AI is a complex process that involves multiple stages. Initially, the system identifies and extracts relevant information from external data sources, such as APIs and databases. This step is crucial for gathering the raw material that will inform the AI’s responses.
Following retrieval, RAG AI undertakes the task of synthesizing this external data with its internal algorithms to generate responses that are not only accurate but also contextually relevant. This phase of information integration is where the true power of RAG AI is manifested, showcasing its ability to transform disparate pieces of data into coherent and comprehensive outputs.
Advancing With RAG AI: The Tangible Benefits
The advancement of RAG AI technology brings with it tangible benefits that extend across various domains. By improving both accuracy and relevance, RAG AI enhances the quality of AI-generated content, making it more applicable and valuable for real-world applications. This leap in performance is particularly significant in fields that rely on precision and up-to-date information, such as healthcare, finance, and legal services.
Moreover, the RAG process itself, by virtue of integrating external data, enables a continuous cycle of learning and improvement. This aspect not only augments the system’s knowledge base but also ensures that AI models remain relevant over time. The ability of RAG AI to adapt and update its responses based on newly acquired information is a game-changer, setting a new standard for what is possible in the realm of artificial intelligence.
How RAG AI Amplifies Large Language Model Memory and Contextualization
Retrieval-Augmented Generation (RAG) AI represents a significant leap in enhancing the capabilities of large language models (LLMs) by amplifying their memory and contextualization through the advantage of RAG. By having the ability to access data from external sources, RAG AI can dynamically incorporate domain-specific data into its responses. This not only broadens the model’s knowledge base but also allows for more precise and contextually relevant outputs, addressing one of the fundamental limitations of standalone LLMs.
Updating Memory and Citing Sources: The Advantages of RAG AI
RAG AI leverages vector databases within its retrieval system to efficiently retrieve relevant documents. This process not only updates the memory of the AI by incorporating the most current information but also enables the model to cite these sources. Such capabilities ensure that the information provided is both accurate and verifiable, enhancing the trustworthiness of AI-generated content.
Moreover, the integration of external data through a sophisticated retrieval system facilitates a continuous learning loop for the AI. As new information becomes available, RAG AI can assimilate these updates, ensuring that its knowledge base remains expansive and up-to-date. This adaptability is crucial for applications requiring real-time data or operating within rapidly changing fields.
Exploring Diverse Approaches and Applications of RAG AI
The versatility of RAG AI is showcased through its diverse applications across various industries. By combining generative capabilities with the accuracy and relevance of information retrieved from multiple sources, RAG AI can generate responses that are not only contextually appropriate but also rich in information. This dual approach ensures that the model has access to a wide range of data, enhancing the quality and reliability of responses generated.
In practical terms, RAG AI’s ability to retrieve relevant content from a plethora of sources before generating responses revolutionizes user interactions. Whether it’s providing customer support, generating personalized content, or aiding in decision-making processes, RAG AI’s nuanced understanding of context and its ability to pull in relevant data make it an invaluable tool across sectors.
The Multifaceted Uses of RAG AI Across Industries
The retrieval system at the heart of RAG AI is a game-changer for multiple industries. By seamlessly integrating with existing databases and the internet, it can pull specific, relevant information in real-time. This capability enables industries such as healthcare, finance, and customer service to provide tailored responses that are informed by the latest, most accurate data available, greatly enhancing the quality of interaction and service provided.
From Chat Applications to Semantic Search: RAG AI in Action
Chat applications leverage RAG AI to deliver responses that are not only contextually relevant but also deeply personalized, significantly improving user experience. Semantic search engines, on the other hand, use RAG AI to understand the intent behind queries, providing results that are more accurate and tailored to the user’s needs. These applications demonstrate the practical benefits of RAG AI in enhancing digital interactions and information retrieval.
Additionally, in environments where precise, up-to-date information is crucial, such as healthcare diagnosis or financial advice, RAG AI’s ability to consult a wide range of external data sources ensures decisions are made based on the most current and comprehensive information available. This not only improves outcomes but also builds trust in AI-driven systems.
Summing Up the Benefits and the Path Forward for Retrieval-Augmented Generation AI
The benefits of retrieval-augmented generation AI (RAG AI) are immense, offering a blend of computational efficiency and enhanced accuracy in generating contextual responses. RAG AI, as a framework, leverages vast volumes of data and sophisticated mathematical representations of data to produce answers that are not only relevant but also original. This AI paradigm significantly reduces the need to retrain the model frequently, thereby saving computational and financial costs. Moreover, by incorporating external knowledge sources such as research papers and databases, RAG AI can cite sources, ensuring data protection and reducing the risk of leaking sensitive data. The path forward involves refining these capabilities to better understand and respond to customer queries, pushing the boundaries of customer service and other applications.
The Role of RAG AI in Fostering Innovation and Ethical AI Development
RAG AI is uniquely positioned to drive innovation across various sectors by providing foundation models with the ability to generate original, contextualized responses. This is achieved by drawing on massive amounts of external data, which enhances the model’s understanding of user queries. Innovation, in this context, comes from RAG AI’s capacity to bridge the gap between generative and retrieval-based models, offering users access to information that is both accurate and up-to-date. Furthermore, by enabling models to cite sources, RAG AI fosters a culture of transparency and accountability, crucial for ethical AI development.
From an ethical standpoint, the development of RAG AI encapsulates the importance of data protection and the measures necessary to prevent the leak of sensitive data. The approach taken by RAG AI, involving the careful integration of external knowledge sources, sets a precedent for handling sensitive information responsibly. Additionally, its application in customer service demonstrates how technology can be used to enhance the user experience while adhering to ethical standards. As RAG AI continues to evolve, its role in promoting both innovation and ethical practices in AI development becomes increasingly significant, marking a new era in how AI interacts with the vast and ever-growing digital world.